Autoencoders#
Summary#
Introduction
Stacked autoencoders
Variational autoencoders
Introduction#
Autoencoders in a nutshell#
Autoencoders are a type of network that aims to encode an input in a latent space and then decode it back.
Autoencoder architecture#
An autoencoder is composed of an encoding function \(E(x)\) outputting a latent representation \(s\), a decoding function \(D(s)\) computing the reconstructed output \(o\) and a loss function \(\mathcal{L}\) measuring the distance between original and reconstructed data.
What’s the point?#
An autoencoder learns to copy its inputs to its outputs under some constraints: for example, limiting the dimensionality of the latent space, or adding noise to the inputs.
To do its job, it must find efficient ways of representing the data: for example, learning the most relevant features and dropping the others.
Latent space properties#
The latent space learned by an autoencoder may have interesting properties.
For example, in a latent space of images of faces, there may be a smile vector \(s\), such that if latent point \(z\) is the representation of a certain face, then latent point \(z + s\) is the representation of the same face, smiling. It becomes possible to add a smile to existing images.
Autoencoders applications#
Dimensionality reduction
Denoising
Data generation
Anomaly detection
Network is trained on normal samples only.
Outliers will induce a high reconstruction loss and will be flagged as anomalies.
Example: performing dimensionality reduction with a linear autoencoder#
(Heavily inspired by Chapter 17 of Hands-On Machine Learning by Aurélien Géron)
Environment setup#
import platform
print(f"Python version: {platform.python_version()}")
assert platform.python_version_tuple() >= ("3", "6")
import numpy as np
import matplotlib
import matplotlib.pyplot as plt
import seaborn as sns
import plotly.express as px
Python version: 3.7.5
# Setup plots
%matplotlib inline
plt.rcParams["figure.figsize"] = 10, 8
%config InlineBackend.figure_format = 'retina'
sns.set()
import tensorflow as tf
print(f"TensorFlow version: {tf.__version__}")
print(f"Keras version: {tf.keras.__version__}")
from tensorflow.keras import Model
from tensorflow.keras.models import Sequential
from tensorflow.keras.layers import Dense, Flatten, Reshape, Layer, Input
from tensorflow.keras.optimizers import SGD
from tensorflow.keras.datasets import fashion_mnist
from tensorflow.keras.metrics import binary_accuracy
from tensorflow.keras.utils import plot_model
from tensorflow.keras import backend as K
TensorFlow version: 2.3.1
Keras version: 2.4.0
Show code cell source
def plot_loss(history):
"""Plot training loss
Takes a Keras History object as parameter"""
loss = history.history["loss"]
epochs = range(1, len(loss) + 1)
plt.figure(figsize=(10, 10))
plt.subplot(2, 1, 1)
plt.plot(epochs, loss, ".--", label="Training loss")
final_loss = loss[-1]
title = "Training loss: {:.4f}".format(final_loss)
plt.ylabel("Loss")
if "val_loss" in history.history:
val_loss = history.history["val_loss"]
plt.plot(epochs, val_loss, "o-", label="Validation loss")
final_val_loss = val_loss[-1]
title += ", Validation loss: {:.4f}".format(final_val_loss)
plt.title(title)
plt.legend()
3D data generation#
np.random.seed(4)
def generate_3d_data(m, w1=0.1, w2=0.3, noise=0.1):
angles = np.random.rand(m) * 3 * np.pi / 2 - 0.5
data = np.empty((m, 3))
data[:, 0] = np.cos(angles) + np.sin(angles)/2 + noise * np.random.randn(m) / 2
data[:, 1] = np.sin(angles) * 0.7 + noise * np.random.randn(m) / 2
data[:, 2] = data[:, 0] * w1 + data[:, 1] * w2 + noise * np.random.randn(m)
return data
x_train_3d = generate_3d_data(60)
x_train_3d = x_train_3d - x_train_3d.mean(axis=0, keepdims=0)
print(f"x_train: {x_train_3d.shape}")
x_train: (60, 3)
# Plot 3D data
fig = px.scatter_3d(x_train_3d, x=0, y=1, z=2, labels={"0": "x1", "1": "x2", "2": "x3"})
fig.show()
Model definition#
dimred_encoder = Sequential([Dense(2, input_shape=(3,))])
dimred_decoder = Sequential([Dense(3, input_shape=(2,))])
dimred_ae = Sequential([dimred_encoder, dimred_decoder])
dimred_ae.summary()
Model: "sequential_2"
_________________________________________________________________
Layer (type) Output Shape Param #
=================================================================
sequential (Sequential) (None, 2) 8
_________________________________________________________________
sequential_1 (Sequential) (None, 3) 9
=================================================================
Total params: 17
Trainable params: 17
Non-trainable params: 0
_________________________________________________________________
Model training#
dimred_ae.compile(loss="mse", optimizer=SGD(lr=1.5))
# Same dataset is used for inputs and targets
history = dimred_ae.fit(x_train_3d, x_train_3d, epochs=20, verbose=0)
plot_loss(history)
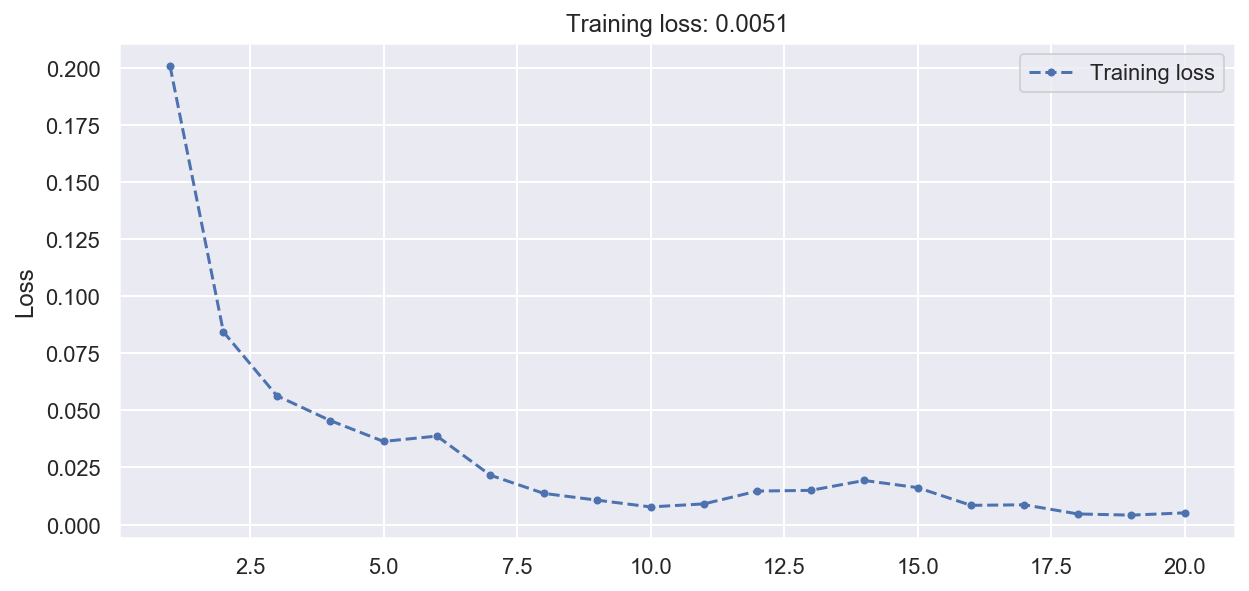
Encoded data representation#
# Plot encoded representation (2D projection) of training data
codings = dimred_encoder.predict(x_train_3d)
fig = plt.figure(figsize=(8, 6))
plt.plot(codings[:,0], codings[:, 1], "b.")
plt.xlabel("$z_1$", fontsize=18)
plt.ylabel("$z_2$", fontsize=18, rotation=0)
plt.show()
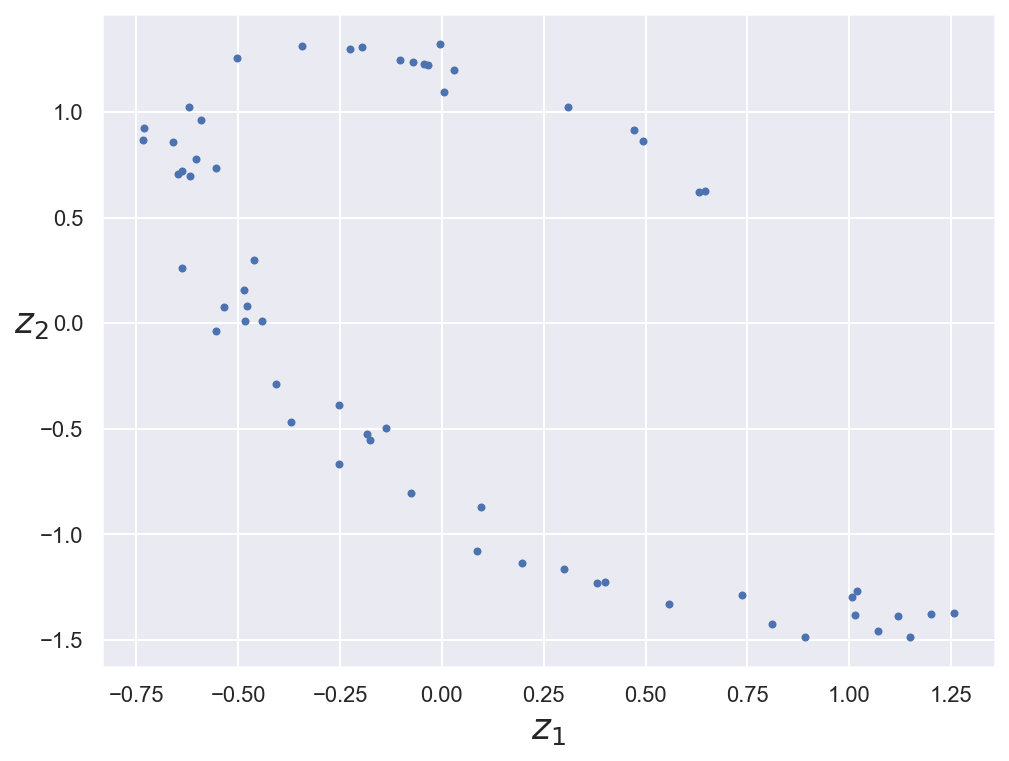
Stacked autoencoders#
Architecture#
Stacked autoencoders have multiple hidden layers and are typically symmetrical. Adding more layers helps them learn more complex codings.
Example: reconstructing fashion images with a stacked autoencoder#
(Heavily inspired by Chapter 17 of Hands-On Machine Learning by Aurélien Géron)
Data loading and preprocessing#
# Load the Keras MNIST digits dataset
(train_images, _), (test_images, _) = fashion_mnist.load_data()
x_train_fashion = train_images / 255.0
x_test_fashion = test_images / 255.0
x_train_fashion, x_val_fashion = x_train_fashion[:-5000], x_train_fashion[-5000:]
print(
f"x_train: {x_train_fashion.shape}. x_val: {x_val_fashion.shape}. x_test: {x_test_fashion.shape}"
)
x_train: (55000, 28, 28). x_val: (5000, 28, 28). x_test: (10000, 28, 28)
The SELU activation function#
Self-normalizing Exponential Linear Unit.
Introduced in a 2017 paper by Klambauer et al.
Can solve the vanishing/exploding gradients problems for deep feedforward networks.
Model definition#
# For each input image, the encoder outputs a vector of size 30
stacked_encoder = Sequential(
[
Flatten(input_shape=(28, 28)),
Dense(100, activation="selu"),
Dense(30, activation="selu"),
]
)
stacked_decoder = Sequential(
[
Dense(100, activation="selu", input_shape=(30,)),
Dense(28 * 28, activation="sigmoid"),
Reshape((28, 28)),
]
)
stacked_ae = Sequential([stacked_encoder, stacked_decoder])
stacked_ae.summary()
Model: "sequential_5"
_________________________________________________________________
Layer (type) Output Shape Param #
=================================================================
sequential_3 (Sequential) (None, 30) 81530
_________________________________________________________________
sequential_4 (Sequential) (None, 28, 28) 82284
=================================================================
Total params: 163,814
Trainable params: 163,814
Non-trainable params: 0
_________________________________________________________________
Model training#
def rounded_accuracy(y_true, y_pred):
"""Define a custom accuracy metric by round predictions"""
return binary_accuracy(tf.round(y_true), tf.round(y_pred))
stacked_ae.compile(
loss="binary_crossentropy", optimizer=SGD(lr=1.5), metrics=[rounded_accuracy]
)
history = stacked_ae.fit(
x_train_fashion,
x_train_fashion,
epochs=20,
validation_data=(x_val_fashion, x_val_fashion),
verbose=0
)
# Plot training history
plot_loss(history)
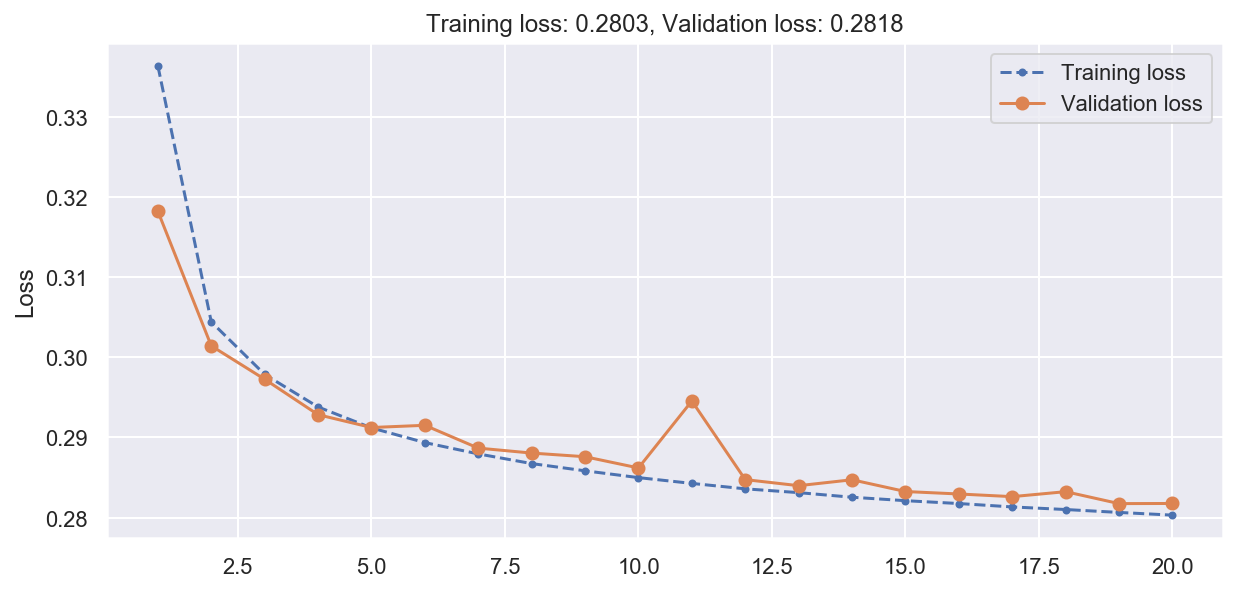
Reconstructions visualization#
Show code cell source
def plot_image(image):
plt.imshow(image, cmap="binary")
plt.axis("off")
def show_reconstructions(model, images=x_val_fashion, n_images=5):
"""Show original and reconstructed images side-by-side"""
reconstructions = model.predict(images[:n_images])
fig = plt.figure(figsize=(n_images * 1.5, 3))
for image_index in range(n_images):
plt.subplot(2, n_images, 1 + image_index)
plot_image(images[image_index])
plt.subplot(2, n_images, 1 + n_images + image_index)
plot_image(reconstructions[image_index])
# Display some validation images with their reconstructions
show_reconstructions(stacked_ae)
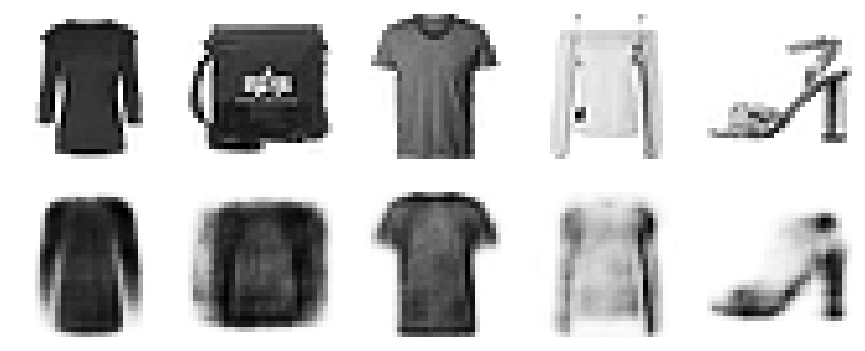
Variational autoencoders#
Principle#
VAE were introduced simultaneously in late 2013 by two research teams (Kingma et al, Rezende et al).
Instead of encoding it into a fixed representation in the latent space, a VAE turns its input into the parameters of a statistical distribution modeling the data: a mean \(\mu\) and a standard deviation \(\sigma\). By sampling points from this distribution, one can generate new input data samples: a VAE is a generative model.
VAE losses#
The parameters of a VAE are trained via two loss functions:
a reconstruction loss forcing the decoded samples to match the initial inputs ;
a latent loss that forces the autoencoder to have codings that look as though they were sampled from a simple Gaussian distribution.
Technically, the latent loss is inplemented as the Kullback-Leibler divergence between the target and actual codings distributions.
\(K\): codings’ dimensionality.
\(\mu_i\) and \(\sigma_i\): mean and standard deviation of the \(ith\) components of the codings.
\(\gamma_i = log_e({\sigma_i}^2)\) is a term introduced for numerical stability.
Example: generating fashion images with a VAE#
(Heavily inspired by Chapter 17 of Hands-On Machine Learning by Aurélien Géron)
Model definition#
class Sampling(Layer):
"""Custom Keras layer to sample the codings (the vector encoding a digit)"""
def call(self, inputs):
# Takes mean and log_var (gamma) as parameters
mean, log_var = inputs
# Sample a random codings vector with same shape as gamma from a Gaussian distribution
# exp(gamma/2) = sigma
return K.random_normal(tf.shape(log_var)) * K.exp(log_var / 2) + mean
latent_dim = 10
# Define encoder model
original_inputs = Input(shape=(28, 28), name="encoder_input")
z = Flatten(name="flattened_input")(original_inputs)
z = Dense(150, activation="selu")(z)
z = Dense(100, activation="selu")(z)
codings_mean = Dense(latent_dim, name="codings_mean")(z)
codings_log_var = Dense(latent_dim, name="codings_log_var")(z)
codings = Sampling()((codings_mean, codings_log_var))
vae_encoder = Model(inputs=original_inputs, outputs=codings, name="encoder")
# Plot non-sequential encoder as graph
plot_model(vae_encoder, show_shapes=True)
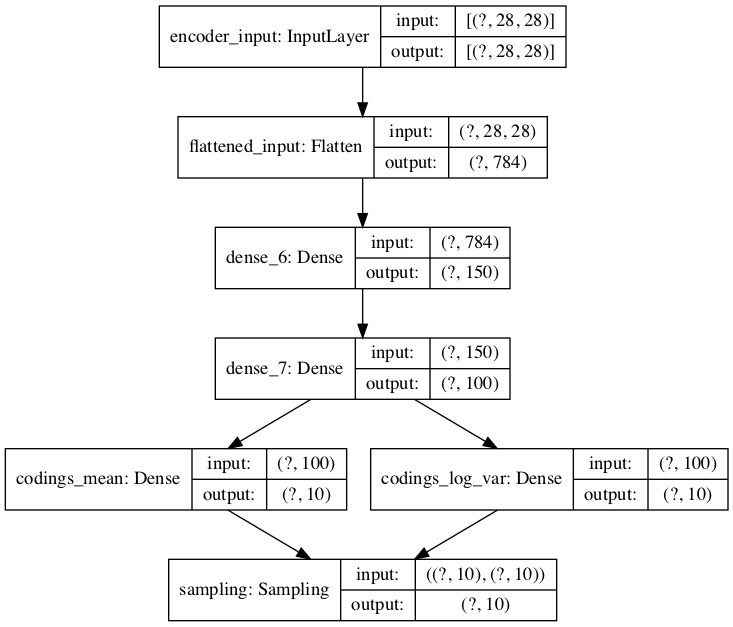
# Define decoder model
latent_inputs = Input(shape=(latent_dim,))
x = Dense(100, activation="selu")(latent_inputs)
x = Dense(150, activation="selu")(x)
x = Dense(28 * 28, activation="sigmoid")(x)
outputs = Reshape((28, 28))(x)
vae_decoder = Model(inputs=latent_inputs, outputs=outputs, name="decoder")
vae_decoder.summary()
Model: "decoder"
_________________________________________________________________
Layer (type) Output Shape Param #
=================================================================
input_1 (InputLayer) [(None, 10)] 0
_________________________________________________________________
dense_8 (Dense) (None, 100) 1100
_________________________________________________________________
dense_9 (Dense) (None, 150) 15150
_________________________________________________________________
dense_10 (Dense) (None, 784) 118384
_________________________________________________________________
reshape_1 (Reshape) (None, 28, 28) 0
=================================================================
Total params: 134,634
Trainable params: 134,634
Non-trainable params: 0
_________________________________________________________________
# Define VAE model
codings = vae_encoder(original_inputs)
outputs = vae_decoder(codings)
vae = Model(inputs=original_inputs, outputs=outputs, name="vae")
# Add KL divergence regularization loss
latent_loss = -0.5 * K.sum(
1 + codings_log_var - K.exp(codings_log_var) - K.square(codings_mean),
axis=-1)
vae.add_loss(K.mean(latent_loss) / 784.)
Model training#
# Train the VAE
vae.compile(optimizer="rmsprop", loss="binary_crossentropy", metrics=[rounded_accuracy])
history = vae.fit(
x_train_fashion,
x_train_fashion,
epochs=25,
batch_size=128,
validation_data=(x_val_fashion, x_val_fashion),
verbose=0,
)
plot_loss(history)
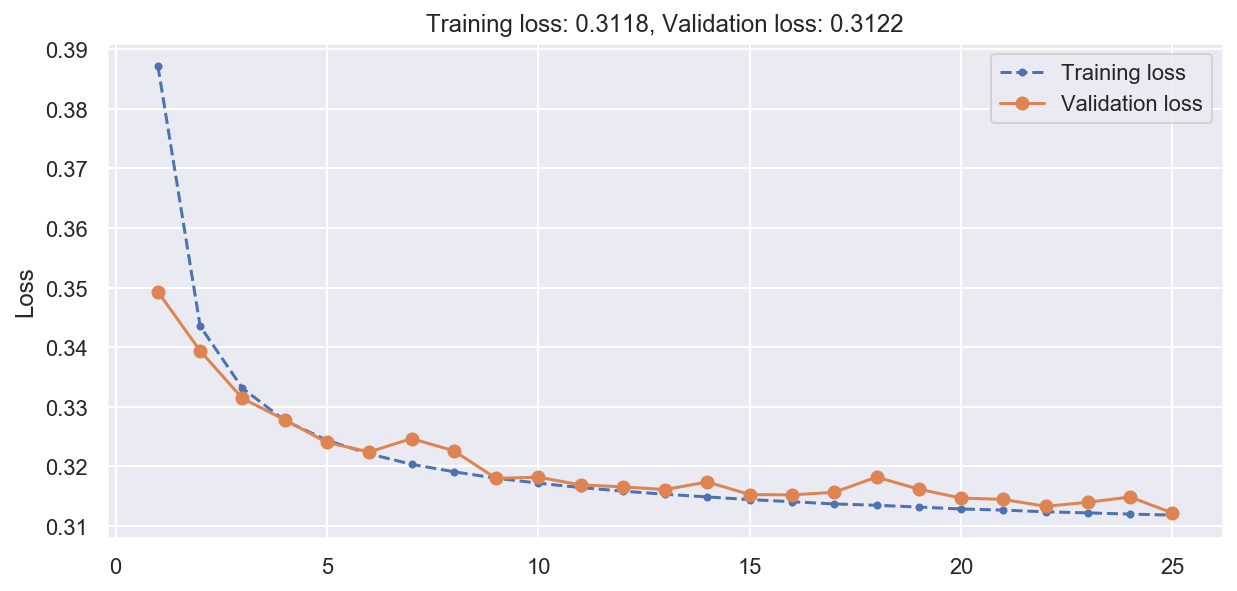
Reconstructions visualization#
show_reconstructions(vae)
plt.show()
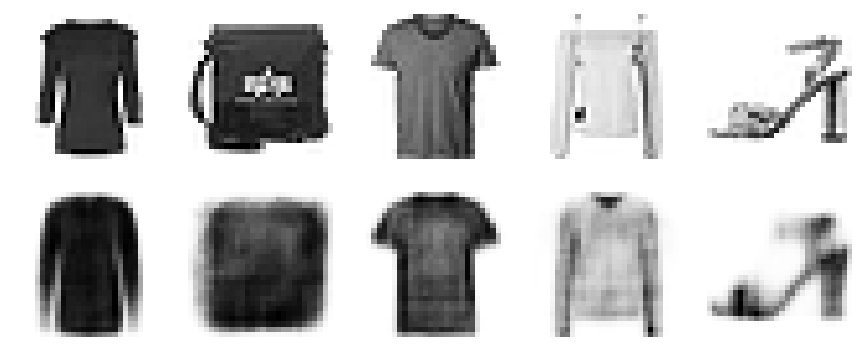
Generating new images#
Show code cell source
def plot_multiple_images(images, n_cols=None):
n_cols = n_cols or len(images)
n_rows = (len(images) - 1) // n_cols + 1
if images.shape[-1] == 1:
images = np.squeeze(images, axis=-1)
plt.figure(figsize=(n_cols*2, n_rows*2))
for index, image in enumerate(images):
plt.subplot(n_rows, n_cols, index + 1)
plt.imshow(image, cmap="binary")
plt.axis("off")
# Generate random codings
codings = tf.random.normal(shape=[12, latent_dim])
# Decode them as new images
images = vae_decoder(codings).numpy()
plot_multiple_images(images, 4)
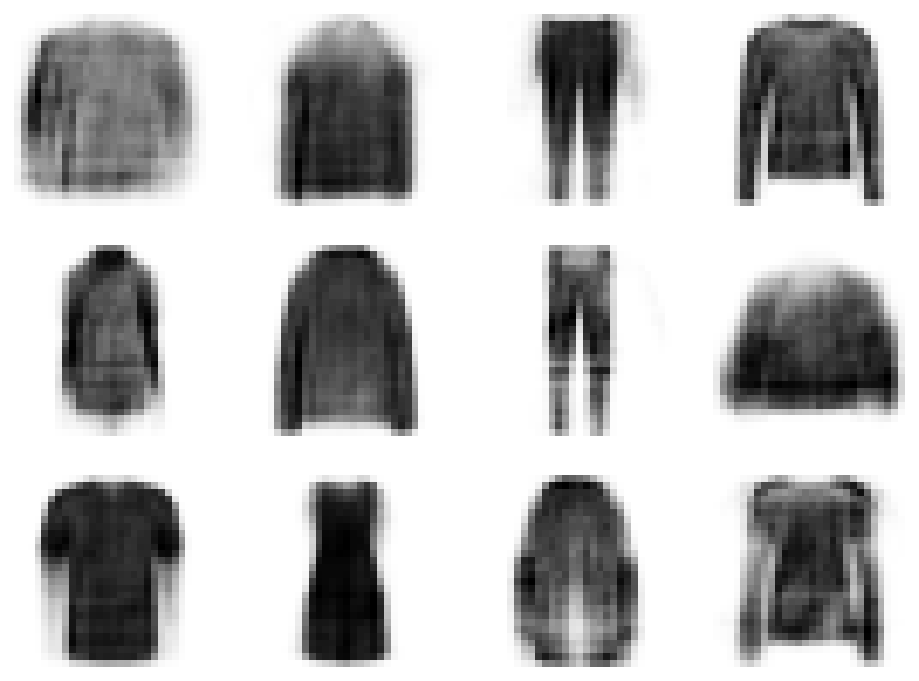